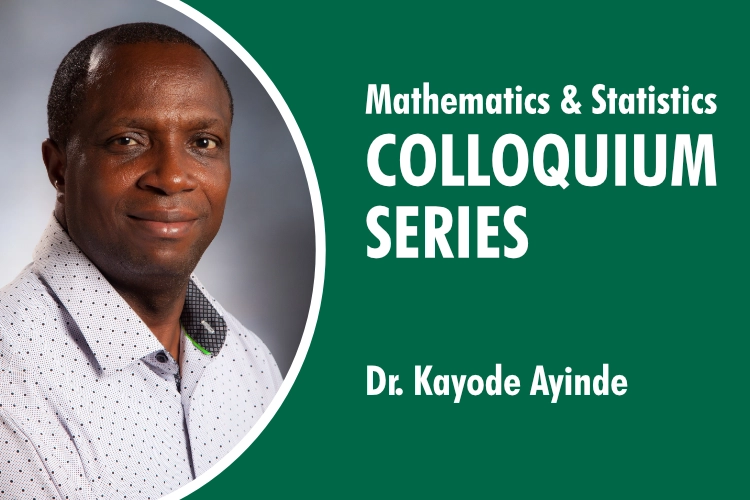
Mathematics and Statistics Colloquium Series #5: Identifying Outliers and Forming Clusters
The Department of Mathematics and Statistics presents the fifth installment of its 2024-25 Colloquium Series, "Identifying Outliers and Forming Clusters: Voting and Principal Component Analysis Techniques with Applications to U.S. Educational and Crime Data" with Assistant Professor Dr. Kayode Ayinde.
The presentation includes a Q&A session, and refreshments will be provided.
Abstract: This research presents new algorithms to form clusters and identify outlier(s) in both univariate and multivariate data sets using the Voting for Most Representative Average (VOMORA) technique. By combining VOMORA with Principal Component Analysis (PCA), the accuracy of outlier detection and cluster formation is enhanced. This method does not only offer a straightforward and comprehensible approach but also serves as an efficient means to summarize and obtain frequency distributions of univariate datasets. The methodology includes determining the optimal number of clusters and organizing the identified clusters in a systematic manner. The techniques are demonstrated with three diverse datasets: USA SAT data (2017-2022), USA Crime data by state (2018-2022), and the iris dataset from the R package. Results categorize U.S. states into three ordered educational clusters and four ordered crime clusters. Improvements are achieved over existing methods, as confirmed by the kappa measure of agreement with the Iris data set and the approach proves to be a valuable tool for data analysis.